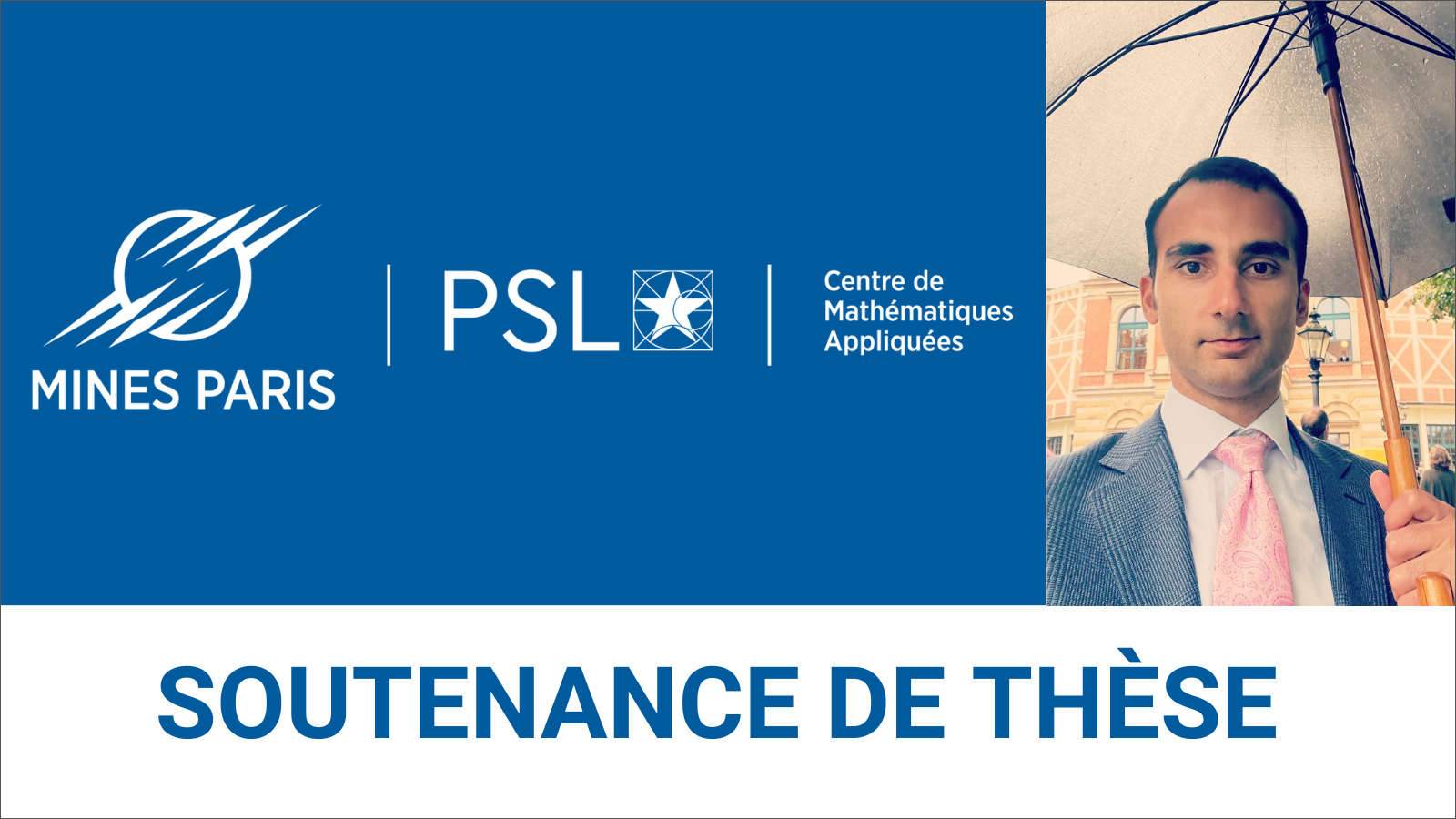
Amirhossein TAVAKOLI soutiendra sa thèse le 20 décembre 2023 à 13h en Amphithéâtre Mozart, Mines Paris – PSL, 1 rue Claude Daunesse, 06560 SOPHIA-ANTIPOLIS
Sujet: Hybrid combinatorial optimization and machine learning algorithms for energy efficient water networks
Sous la direction de : Sophie DEMASSEY (CMA Mines Paris – PSL) et Valentina SESSA (CMA Mines Paris – PSL)
Cette thèse a bénéficié d’un financement de l’Institut 3IA Côte d’Azur
Abstract : Drinking water distribution networks are energy-intensive systems, mainly due to pumping. However, they offer opportunities for load reduction and shifting, thanks to water towers and their storage capacity. Optimized operational management of pumping and storage in water networks, also known as “pump scheduling”, is therefore an advantageous lever for electricity networks, but it is also a complex mathematical optimization problem. The object of this thesis is the design of efficient resolution algorithms for a detailed, discrete and non-convex mathematical model. Unlike most of the literature on the subject, the emphasis is placed on the calculation of strictly feasible, possibly optimal, solutions of the mathematical model. Furthermore, the study strives to mitigate the algorithmic complexity of the problem due specifically to the coupling storage constraints, and presents different approaches to operate and exploit the temporal and spatial decomposition of the model.
A first contribution thus concerns the design of preprocessing techniques for bound tightening and cut generation. Bounds and cuts are obtained from detailed partial (on a truncated time horizon or a part of the network) relaxations, and make it possible to reinforce a simpler (continuous and linear) general relaxation, basis of a global optimization algorithm. A second contribution concerns the development of an original local optimization algorithm, of the “Alternating Direction Method” type, which progressively refines a storage profile until reaching the associated valid pump schedule. Indeed, by fixing the coupling storage constraints at each iteration, the restricted non-convex discrete model can be solved exactly, by temporal and spatial decomposition. The algorithm thus recovers a feasible solution (a pumping plan) from a near-feasible near-optimal solution (a storage profile). If many heuristics from the literature can be invoked to generate this initial solution, we propose to obtain it by building a data model. The third contribution of the thesis thus concerns the development of a deep learning model, relying on the history of operations of a given network, and resulting in a data model complementary to the hybridized mathematical model. Scalability is an original feature of the approach, making it possible to learn a solution with a fine temporal discretization from a dataset for a coarse temporal discretization, thus remedying the difficulty of dataset generation. Finally, note that this hybrid combinatorial optimization and machine learning algorithm applies to other discrete optimal control problems of systems with storage. The empirical evaluation went through the generation of extensive training and experimentation datasets on networks from the literature and highlighted the performance of the exact and approximate algorithms.
Mots clés : mathematical optimization – machine learning – water networks
La soutenance se tiendra en anglais et sera également retransmise en directe. Pour recevoir le lien de connexion, merci de nous contacter.