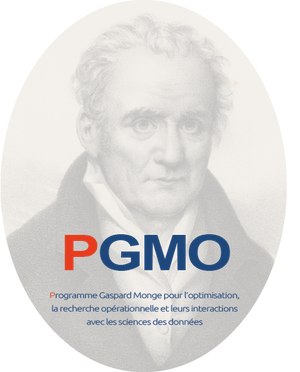
PGMO IROE : Models for planning energy investment under uncertainty
Responsable du projet au CMA
Type de projet
PGMO – Programme Gaspard Monge pour l’Optimisation, la recherche opérationnelle et leurs interactions avec les sciences des données (Fondation Mathématique Jacques Hadamard)
Partenaires
• Felipe Atenas, Unicamp (Brésil)
• Wim van Ackooij, EDF R&D OSIRIS (France)
• Rafael Correa, State University of O’Higgins (Chili)
• Claudia Sagastiz´abal, Unicamp (Brésil)
Description du projet
To succeed in the transition to sustainable power systems, it is of major importance to improve investment strategies and operational planning of energy supplies. Intermittent sources of energy are changing the traditional electricity systems. State-of-the-art research in the area must take into account new considerations, such as minimizing carbon emissions, the development of efficient production technologies and storage at small scale, and various technological changes in instrumentation and communication. The project considered generation expansion models under these new paradigms, with the objective in the long term to embed them into multiple leader-follower problems. Generation expansion problems determine the most beneficial increase in the productive capacity of an electric energy system as a whole. The objective is to maximize the overall social welfare and to minimize the investment costs incurred in building production facilities. To guarantee that generating units efficiently and reliably satisfy demand, it is necessary to invest in building new electricity production facilities or to expand the capacity of the existing generating units. In the presence of intermittent sources of energy, uncertainty in the generation expansion problem can be addressed by means of a multistage stochastic program with risk aversion. In this projected, we have investigated theoretical properties of the model as well as solution techniques suitable for the structure of the optimization problem.
Publications dans le cadre du projet
Publications in scientific journals
1. M. CORDOVA, W. DE OLIVEIRA, AND C. SAGASTIZÁBAL, Revisiting augmented lagrangian duals, Math. Prog., 2022.
2. W. DE OLIVEIRA. Risk-averse stochastic programming and distributionally robust optimization via operator splitting, Set-Valued and Variational Analysis, Volume 29, pp. 861–891, 2021.
3. F. BELTRÁN, E. C. FINARDI, AND W. DE OLIVEIRA, Two-stage and multi-stage decompositions for the medium-term hydrothermal scheduling problem: a computational comparison of solution techniques, International Journal of Electrical Power and Energy Systems, (2021).
4. F. BELTRÁN, E. C. FINARDI, G. M. FREDO, AND W. DE OLIVEIRA, Improving the performance of the stochastic dual dynamic programming algorithm using chebyshev centers, Optimization and Engineering, (2020).
5. B. COLONETTI, E. C. FINARDI, AND W. DE OLIVEIRA, A mixed-integer and asynchronous level decomposition with application to the stochastic hydrothermal unit-commitment problem, Algorithms, 13 (2020), p. 235.
6. W. DE OLIVEIRA, The ABC of DC programming, Set-Valued and Variational Analysis, 28 (2020), pp. 679–706.
7. W. DE OLIVEIRA, Sequential difference-of-convex programming, Journal of Optimization Theory and Applications, 186 (2020), pp. 936–959.
8. M. FUKUSHIMA, J. JÚDICE, W. DE OLIVEIRA, AND V. SESSA, A sequential partial linearization algorithm for the symmetric eigenvalue complementarity problem, Computational Optimization and Applications, 77 (2020), pp. 711–728.
9. W. VAN ACKOOIJ, W. DE OLIVEIRA, AND Y. SONG, On level regularization with normal solutions in decomposition methods for multistage stochastic programming problems, Computational Optimization and Applications, 74 (2019),
pp. 1–42.
10. W. VAN ACKOOIJ, S. DEMASSEY, P. JAVAL, H. MORAIS, W. DE OLIVEIRA, AND B. SWAMINATHAN, A bundle method for nonsmooth DC programming with application to chance-constrained problems, Computational Optimization
and Applications, (2020).
Chapter of book
11. W. DE OLIVEIRA AND M. SOLODOV, Bundle methods for inexact data, in Numerical Nonsmooth Optimization, Springer International Publishing, 2020, pp. 417–459.