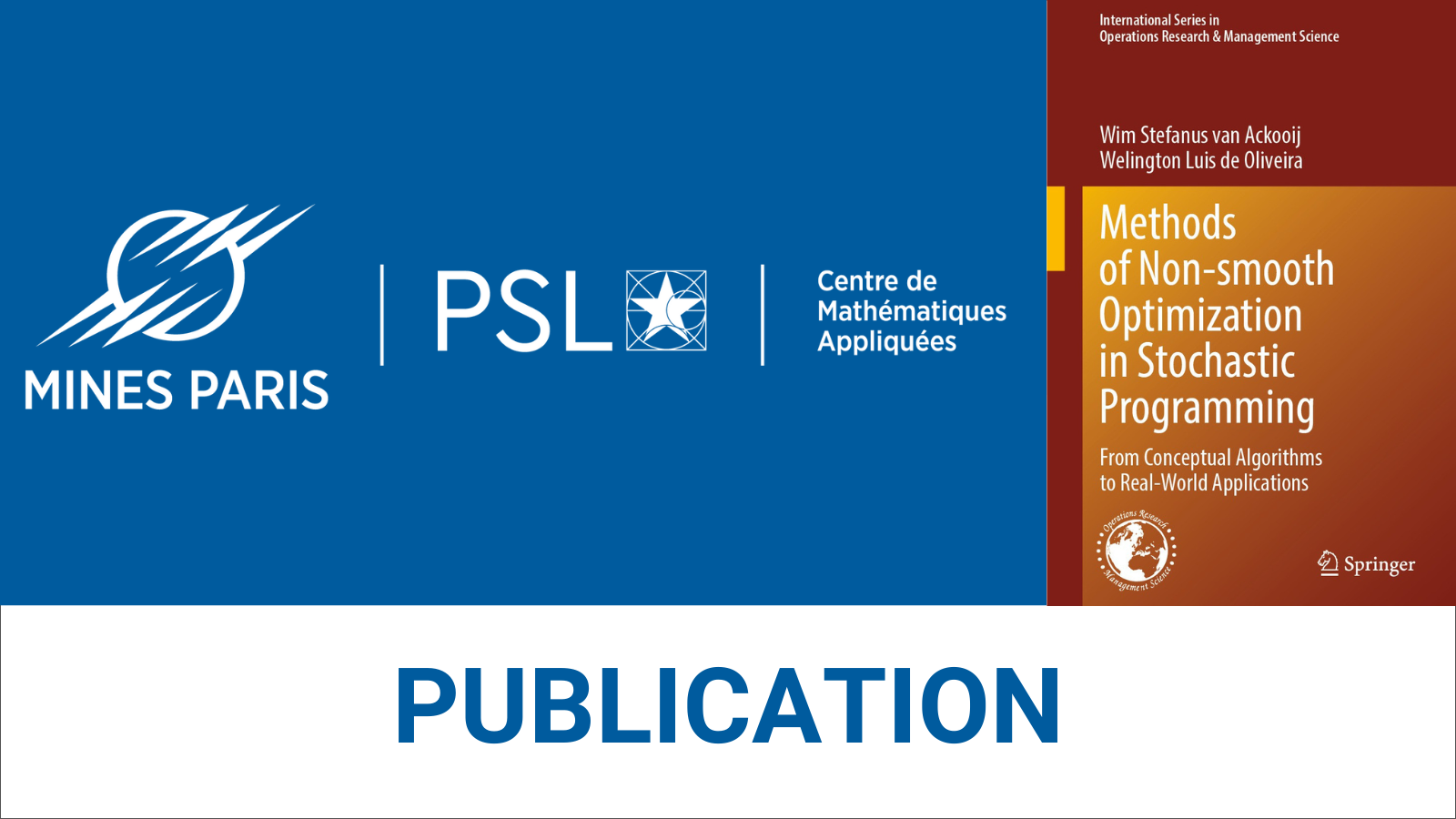
Publication aux éditions Springer Cham de l’ouvrage Methods of Nonsmooth Optimization in Stochastic Programming par Welington DE OLIVEIRA (CMA Mines Paris – PSL) et Wim VAN ACKOOIJ (EDF)
Cet ouvrage :
- Présente des méthodes avancées d’optimisation non lisse et de programmation stochastique
- Offre des conseils étape par étape avec une analyse mathématique rigoureuse
- Introduit des algorithmes pratiques et implémentables
Résumé :
This book presents a comprehensive series of methods in nonsmooth optimization, with a particular focus on their application in stochastic programming and dedicated algorithms for decision-making under uncertainty. Each method is accompanied by rigorous mathematical analysis, ensuring a deep understanding of the underlying principles. The theoretical discussions included are essential for comprehending the mechanics of various algorithms and the nature of the solutions they provide—whether they are global, local, stationary, or critical. The book begins by introducing fundamental tools from set-valued analysis, optimization, and probability theory. It then transitions from deterministic to stochastic optimization, starting with a thorough discussion of modeling, understanding uncertainty, and incorporating it into optimization problems. Following this foundation, the book explores numerical algorithms for nonsmooth optimization, covering well-known decomposition techniques and algorithms for convex optimization, mixed-integer convex programming, and nonconvex optimization. Additionally, it introduces numerical algorithms specifically for stochastic programming, focusing on stochastic programming with recourse, chance-constrained optimization, and detailed algorithms for both risk-neutral and risk-averse multistage stochastic programs. The book guides readers through the entire process, from defining optimization models for practical problems to presenting implementable algorithms that can be applied in practice. It is intended for students, practitioners, and scholars who may be unfamiliar with stochastic programming and nonsmooth optimization. The analyses provided are also valuable for practitioners who may not be interested in convergence proofs but wish to understand the nature of the solutions obtained.
Mots clés :
Nonsmooth optimization, Stochastic programming, Numerical optimization, Optimization under uncertainty, Set-valued analysis, Algorithms, Numerical algorithms, Nonconvex nonsmooth optimization, Stochastic optimization, Mixed-integer convex programming, Convex optimization, Risk-averse multistage stochastic programs